Personalized Recommender.
Have you ever wondered how amazon is showing you the product that you did not even think of making a purchase before looking at the product? Have you ever seen an unheard-of movie on Netflix and ended up liking it? How are they able to predict what you did not even know you will like it? With advent of deep learning methodologies and availability of large data, neural network-based recommendation systems have emerged as an important tool for personalization task in eCommerce websites.
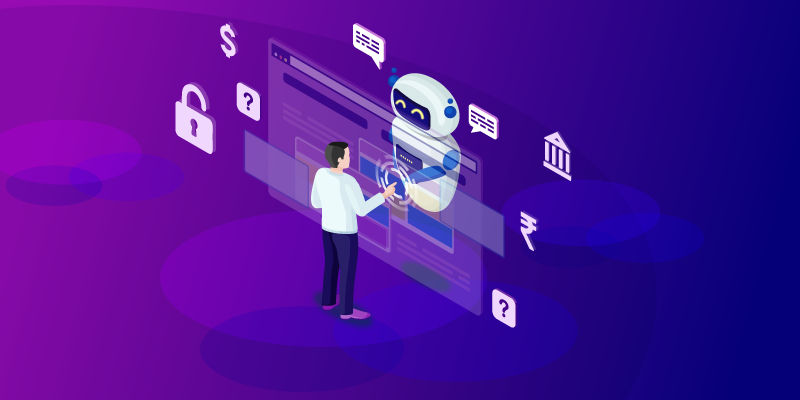
The answer to the question is state of the art recommendation engines. Recommendation engines are open to scale machine learning algorithm that are exploited in almost all consumer product purchases like ecommerce, video streaming, gaming and even in matrimonial services. There are a lot research and work that has gone into development of precise recommendation engines for large scale product to customer matching.
Some of the more familiar recommendation approaches are: collaborative filtering, matrix factorization, singular vector decomposition, and matrix factorization. Our team has worked in development of recommendation engines for improving click through rate (CTR) as well as to improve the conversions on the website to boost revenue or other metrics on the website.
With growing traffic on the web pages, the recommendation systems are not essentially a univariate data point of the user action, rather a very complex set of actions that are performed over a period of time on the website. With our recommendation systems, from a large number of available choices for items or services, we can present the customers with much narrower list of items the customers are likely to engage with.
Recommendation engines has lot of applications, some of them are:
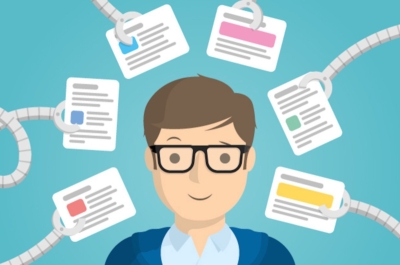
AiNxt leverages few of the markets leading recommendation systems algorithms like matrix factorization and ALS, which have proven to increase selected metrics on the customer journey on the website. We can customize a tailor-made recommendation engine for your product needs with our data scientist who have spent considerable amount of time building multiple recommendation engines.